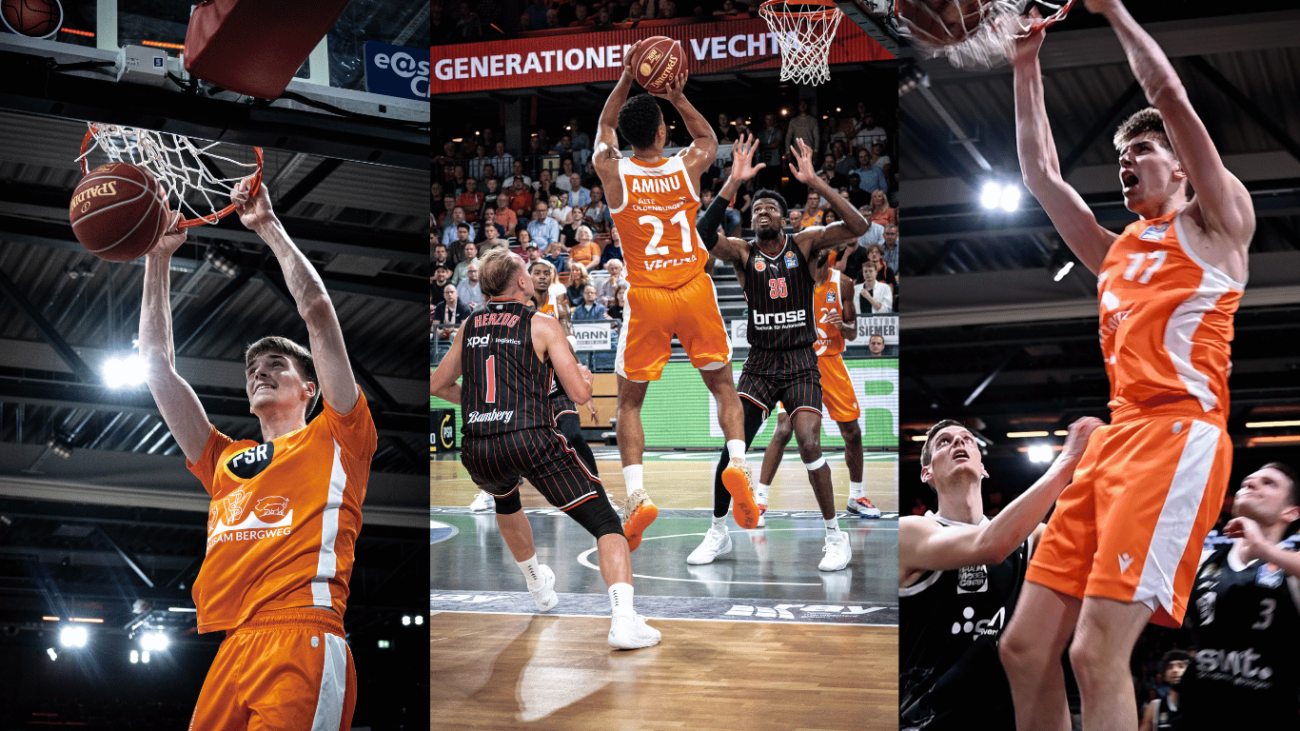
This article is written by Domenik Theodorou, a Firstbeat Sports client and Performance Coach at RASTA Vechta, a professional basketball team competing in the Basketball Bundesliga (BBL). Before his current role, Domenik also worked at Bamberg Baskets and BG Göttingen. In this article, Domenik shares practical advice and tips on using Firstbeat to help identify trends in player fitness.
In this blog post, I will explain how we can employ different Firstbeat parameters to recognize and track players’ fitness trends from the pre-season until the end of the competition.
Let’s take the pre-season as an example. Our preparatory period in 2023 lasted 6 weeks and included 20 team practices, 15 individual workouts, 12 lifting sessions, and five friendly matches. If a player makes it through this period without sustaining an injury, I think it is safe to say that his physical condition will be better than it was at the start of the pre-season. At least that’s what my instincts told me in the past when I didn’t have access to an athlete performance management platform like Firstbeat. I had to rely on the eye test and intelligent guessing only.
What are my key parameters for fitness trend recognition?
If you collect data consistently and always in the same manner, you can compare it with previous collections. Our players wear the Firtbeat sensors during team practices, individual workouts, and shooting sessions. The goal is to capture everything that happens on the court besides games.
I like to use TRAINING IMPULSE (TRIMP), MOVEMENT LOAD (ML), MOVEMENT EFFICIENCY (ME) and HIGH INTENSITY TRAINING (HIT) for fitness trend recognition. ME describes the relationship between internal and external load and is simply calculated by dividing ML by TRIMP. This is not an official parameter provided by Firstbeat but it is still an interesting ratio to follow.
I look at ME particularly during team practices. I would like to show you an example of a player who played multiple years for our team. This is very convenient because it allowed me to compare the data from the first and second seasons playing for our club. By comparing data from different phases of two seasons, I can get much more insights into the conditioning state of the player than just with one season. I would also like to point out that you need to obtain an accurate MAX HR for each player on your team because otherwise, the data collection won’t be realistic.
How to organize data to recognize fitness trends?
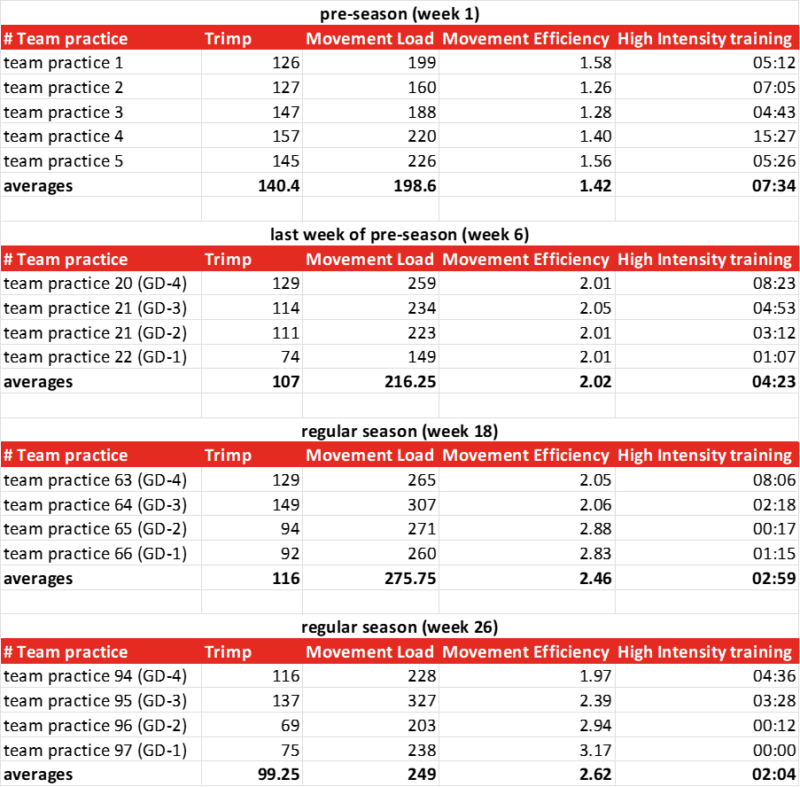
Figure 1
In Figure 1 you can see practice loads at different points of time from the player I mentioned above. This is a snapshot of how I set up the data for each player on a daily basis. If you look at the first week of pre-season it is important to note that we intended to keep the practices short without including any contact drills.
We wanted to give the players a chance to adapt and recover between sessions. Keeping these two aspects in mind, I think it is very interesting to observe that the player averaged a very high TRIMP in relation to the relatively low ML, hence showing a low ME. This also explains the high number of minutes he spent above 90% of his MAX HR.
Studying the later phases of the season you can recognize a trend. It looks like the athlete can do more basketball-specific work at a lower internal cost. The different examples show a tendency for lower TRIMP, and fewer minutes of high-intensity training while being exposed to a higher ML which leads to improved ME.
This tells me that the players’ conditioning, the ability to maintain strength, power, and speed across practice or competition, has improved. The athlete seems to be able to delay the point at which the body produces energy predominantly throughout the anaerobic pathways.
In other words, the player can rely longer on his aerobic system to produce energy. This is closely connected with a better ability to recover between intense bouts of exercise. His body has simply become more efficient in meeting the demands of basketball and in dealing with metabolic byproducts. His work capacity has improved because he can do more work while experiencing less fatigue.
As I mentioned in the beginning, this is a development you might expect anyway throughout the course of a competitive season. Without monitoring, I would only be able to guess such an improvement. I strongly believe that a little bit of direction in chaotic environments allows you to be more flexible and adaptable. In professional sports, you constantly look for signals.
If I had to choose between being able to use a monitoring system or working without it, at this point, I would always opt for data collection.
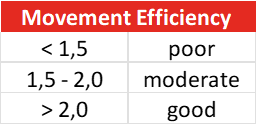
Figure 2
Figure 2 gives you a little bit of direction on how to interpret ME in basketball. This solely goes back to my personal experience gathering Firstbeat data over the last three years. It is a very simple attempt on how to evaluate ME.
How to compare data?
We must keep in mind that we should be careful comparing basketball practices because they will always be different. Practice weeks will also vary as you can see by comparing week 18 to week 26 in Figure 1.
You will also see big interindividual differences among players. Some players accumulate TRIMP and ML at a different rate than others. This is why you should focus primarily on intraindividual comparisons because each athlete has their own physiological signature. Comparing the same player gives you chances to recognize trends or red flags that you couldn’t spot by only relying on the eye test or your intuition.
Let’s assume our player from Figure 1 suddenly shows a ME of 1,4 during the second practice of week 33. As a next step, I would look at the rest of our team to find out if they also show a meaningful change to their usual workload ranges. If that is not the case, I have good reason to approach our player to find out if everything is ok.
This is exactly where the use of a tool like Firstbeat comes into play. Knowing the training data for each of our athletes simply enables us to ask better questions and complements decision-making. It also helps in understanding how our athletes are coping with the program.
Please keep in my mind that this is only my way of organizing the Firstbeat data to get a better understanding of the athletes I work with. I’d be very interested in other ways of maximizing Firstbeat. If you have questions or remarks or simply want to share your way of implementing the system, don’t hesitate to reach out to me.
Get more insights from Domenik by watching our webinar recording on Mastering Data-Driven Decisions in Pro Basketball here.
If you liked this article, you should subscribe to our newsletter.
You might also be interested in
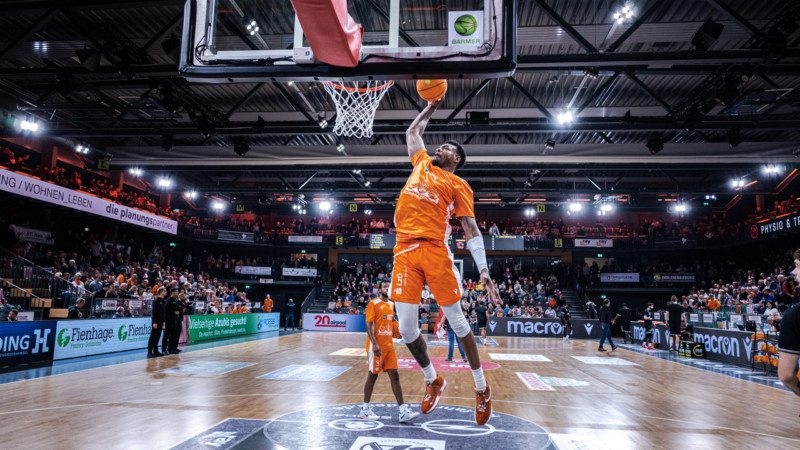
Shooting For The Top Again with RASTA Vechta and Firstbeat Sports
RASTA Vechta is a basketball team currently playing in the Pro A, the second division of professional basketball in Germany. We recently spoke with Vechta’s Performance Coach, Domenik Theodorou, to…
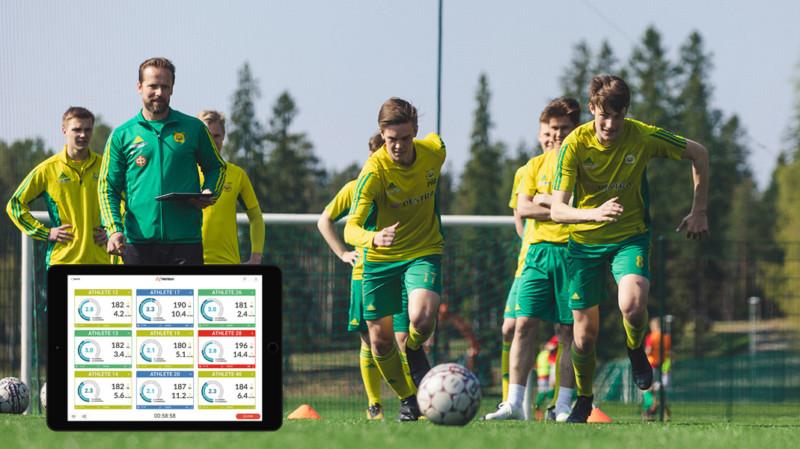
Movement Load: Add Valuable Context to Internal Load Data
Movement Load metric allows you to quantify the movement of your athletes using the Firstbeat Sports platform. But what exactly is Movement Load? What should you do with information it…